Weird Data Science
What goes into creating an artificially intelligent platform that is capable of making decisions? Rule-based machine learning? The Three Laws of Robotics? A copy of Cryptosmasher v3.10 and an Oingo Boingo soundtrack? Join Red Hat CTO Chris Wright and Sherard Griffin in this episode as they explore artificial intelligence and machine learning's relationship to data, the role that open source plays in the development of models, and how projects like Open Data Hub can foster a culture of innovation.
00:02 — (notification sound)
Ah, nice. I don't know about you, but I'd miss half my meetings without automated calendar notifications. It's the only way to cope with an overloaded to-do list. I guess the age-old tactic is just drink more coffee. Wouldn't it be nice if my coffee maker could actually read my calendar and know to make another cup? Or better yet, read my facial expressions? And know to make it stronger? But for that, we'd need AI.
00:35 — INTRO ANIMATION
Where automation can only follow the instructions that humans provide. AI is a larger concept for creating intelligent machines that simulate human thinking and behavior to execute tasks smartly. In other words, AI encompasses machine learning. Machine learning is a subset of AI that allows machines to learn from data without explicit programming. Machine learning algorithms adapt themselves to perform better as they are exposed to more data. And the ML model represents what's been learned by a machine learning algorithm. Every one and their grandmother is a consumer of AI. Whether you shop online, browse recommended videos or run search queries, AI platforms use data to determine what's most relevant. The pace and growth of AI technology is already making a lasting impact on the world. Actually, I have a few examples bookmarked. Let's see. Oh, um, I don't know if it's quite ready, but this might be fun. Hat-E, can you show me a few cool AI use cases?
The Royal bank of Canada created the Borealis AI platform with the ability to run thousands of simulations and analyze millions of data points to deliver next-generation banking applications.
All right. Not too bad. What else have you got?
HCA Healthcare created a real-time predictive analytics product system to more accurately and rapidly detect sepsis, a potentially life-threatening condition.
I'm actually pretty impressed. Ah, Sherard. Let's see. What? Oh, wait that's not... Wait, what's happening?
You seem to have too many items on your calendar, Chris. I think you would benefit from some resting cycles.
Oh no, no come on. Hat-E, I think you need some more training. Your sentiment analysis is working well but your next recommended action, we need to work on it a little bit.
No Chris, no-
Hey Chris, I just saw canceled meeting on my calendar. Do you need to reschedule?
Oh man sorry. My bad, long story it's kind of an AI thing gone awry but I've been thinking about transparency and understanding the decisions that AI models make. Means you have to understand the data that goes into those AI models and in there there's a whole discussion around bias, but I really just want to understand your thoughts around the role that open-source plays in the AI model development.
Yeah, I, I'm actually really glad you've been thinking about that, Chris, because it is an area that we've been focusing on. If you look at the model without the data, then it's very hard to understand the decisions that an AI application may have made, but then if you have the data, but no model, then you don't understand the innovation that went into the model itself. And then of course at the very end of it, if you don't have open-source tools that allow you to create and edit and, and, and really iterate over the process over and over again. You know, without any one piece of that, you have a disconnected story. In an Open Data Hub, what we're doing is bringing all of those things together: the data, the tools, the models and just doing it in a way that Red Hat knows best, which is in an open source community-based environment.
I really like that. I mean, I'm drawing an analogy in my mind about open source development and software and a lot of visibility that goes in that software development process. AI needs the same thing: data as the source, building the models, using open source tools. And I think one of the cool things around Open Data Hub is you can actually surface a model as a microservice available to application developers and really bring that all together from that innovation point of view.
Now what we're looking at is how do we apply that same fundamental concept but now move it over into AI and ML. And so, when we look at ways in which we needed to do this, it really centered on what we know best, which is democratization and making things freely available for people to use.
I have to admit, I always get a little hung up on the word democratization because it feels a little bit like a cheesy marketing slogan but the way you're using it here is actually a perfect use, dictionary definition use of the word democratization, which is really about leveling the playing field and ensuring everybody has access because, that broad access creates fundamentally better outcomes.
It's about openness. It's about collaboration. When we bring all of those things together: the data, the models, the tools and it's all done in an open, innovative way, we're actually fueling innovation and creativity and allowing organizations to create things at a rapid pace.
Sherard, I'm glad we kept the time on the calendar and, the reason I'm thinking about this transparency and models is, the stakes are really high. When we think of things like autonomous vehicles or medical recommendations where, literally, it's a matter of life and death. So we really have to shine a light on the process of data and training and building models. So thanks a lot, Sherard.
Absolutely.
Data is crucial to machine learning models and those models are critical to using compute power to gain insights and create innovation. Transparency into how data is used to train AI is not only important for technologists developing next generation applications, but also for consumers. So they can be part of innovation. Think about what's possible with more equitable open data. That's why I like to say: open unlocks the world's potential.
06:56 — OUTRO ANIMATION
- Keywords:
- AI/ML
Meet the guest
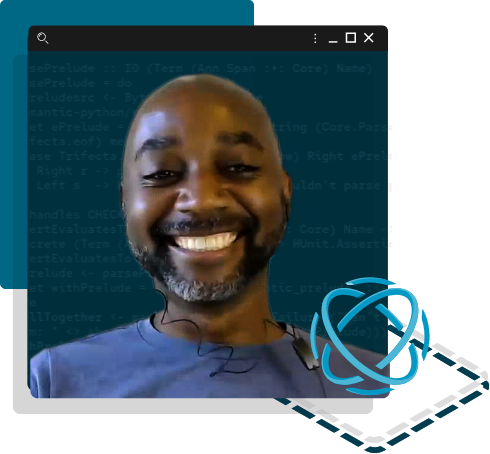
Sherard Griffin
Senior Director of Engineering
Artificial Intelligence Services
Red Hat
Keep exploring
Accelerate ML pipelines and intelligent app delivery
Discover how Red Hat and our certified partners provide solutions to accelerate AI/ML initiatives with confidence.
Explore our AI partner ecosystemHow to start your AI/ML journey
Some organizations have implemented machine learning technology but have not seen the expected return on their investment. So what can you do to help ensure success with your AI/ML investment?
Read the blog postMore like this
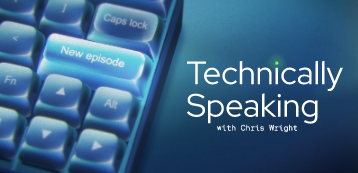
Machine Learning Model Drift & MLOps Pipelines
Like houseplants, machine learning models require some attention to thrive. That's where MLOps and ML pipelines come in.
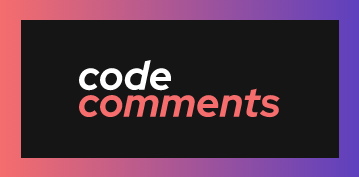
Bringing Deep Learning to Enterprise Applications
To realize the power of AI/ML in enterprise environments, users need an inference engine to run on their hardware. Two open toolkits from Intel do precisely that.
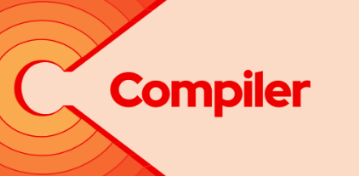
How Do Roads Become Smarter?
Smart road technology can make travel safer, easier, and more efficient. But how can it make travel enjoyable?
Share our shows
We are working hard to bring you new stories, ideas, and insights. Reach out to us on social media, use our show hashtags, and follow us for updates and announcements.
Presented by Red Hat
Sharing knowledge has defined Red Hat from the beginning–ever since co-founder Marc Ewing became known as “the helpful guy in the red hat.” Head over to the Red Hat Blog for expert insights and epic stories from the world of enterprise tech.